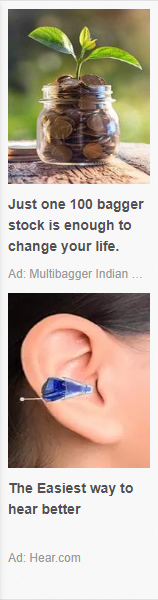
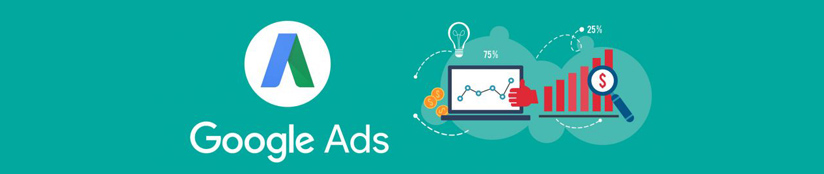
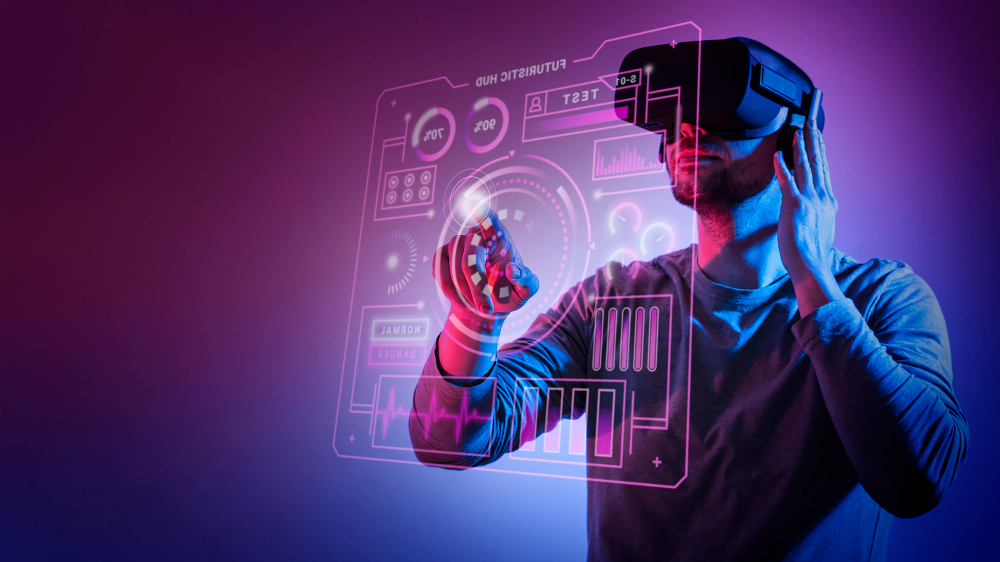
The Difficulties Australian Organizations Face in Illustrating AI ROI
Australian organizations are at a pivotal point in the quickly changing AI world.
AI clearly has the potential to bring about large financial gains; according to some reports, implementing an AI portfolio can result in additional EBITDA of over $100 million. However, achieving ROI is not without its difficulties.
Up to 85% of enterprise AI installations fall short of business expectations. The hazards associated with AI are highlighted by its high failure rate, which even outpaces the well-known challenges of earlier attempts at digital transformation.
The consequences of an AI deployment gone wrong could be disastrous. Australia is a prime example of the risks associated with AI, as demonstrated by the “Robodebt” crisis, which hurt Australians so much that a Royal Commission was formed to look into it.
A Gartner analyst provides guidance.
While many people are enthusiastic about the opportunities presented by AI, surveys reveal that 80% of Australians are extremely worried about the threats the technology poses and believe these concerns should be given “global priority.”
However, CIOs are investing heavily in AI initiatives in spite of the risks and social reluctance; according to KPMG data, over half of Australian businesses allocate between 10 and 20 percent of their budget to AI.
The CIO and IT staff will have even more responsibility to make sure AI programs pay off. To make AI a sustainable investment possibility, organizations need to get past their risk apprehensions. According to research from Gartner, the biggest obstacle to AI projects is determining and proving business value.
According to TechRepublic, Gartner’s senior director analyst in Finance Technology, Value, and Risk, Nate Suda, many organizations struggle to articulate the value of artificial intelligence (AI). These challenges include cost management, productivity gains, and the strategic approaches required to guarantee that AI investments result in real business value.
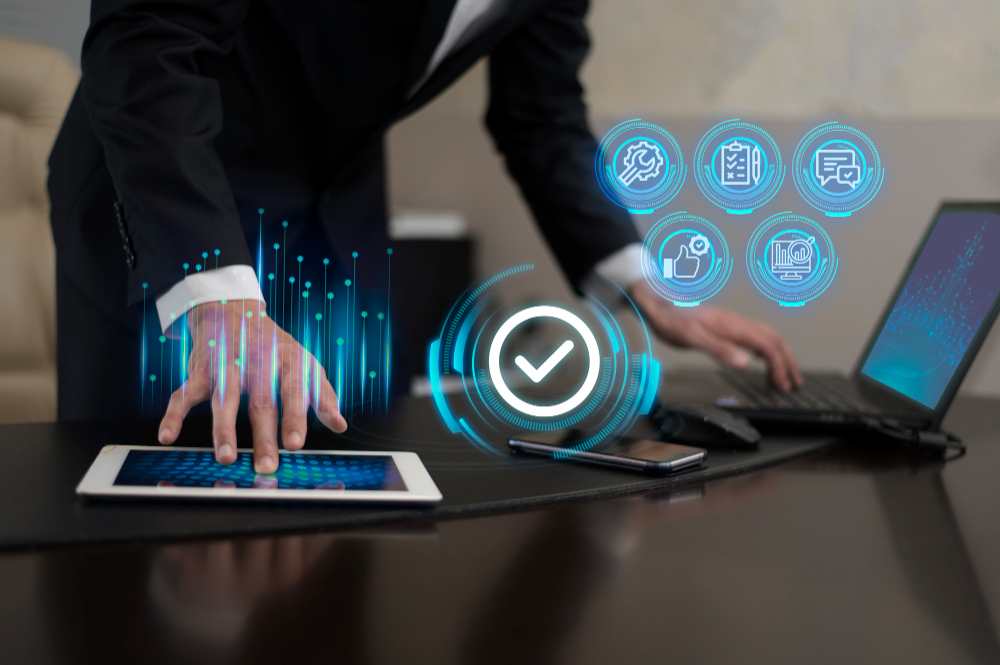
Comprehending cost dynamics
The main obstacle to AI implementations is cost management. Generic artificial intelligence (generative AI) is more expensive than standard search engines since it is interactive.
To fine-tune their comments, users sometimes participate in repeated exchanges, which dramatically raises costs. The cost increases with each interaction, which is expressed in tokens. Should user behaviour diverge from early expectations, this expense could soar.
According to Suda, “Human interaction is one of the biggest variables in cost.” You can’t just type in a question and obtain a flawless answer when using generative AI. It may take multiple tries, and each word of your inquiry and answer will be paid for. Your costs may increase significantly if users engage in several interactions but your cost model only accounts for one.
Organizations are implementing a “slow scale-up” strategy in order to reduce this risk. They first execute the planned AI deployment with a small number of users before progressively expanding the number of users, as opposed to a quick, large-scale deployment.
By using an iterative approach, businesses can monitor the progress of ambitious AI initiatives and make adjustments based on real usage patterns, enabling them to more correctly forecast costs and prevent unpleasant surprises.
According to Suda, “the best organizations are scaling up very slowly.” “In the first month, they might have 10 users, then 20 in the next, and so on. This approach aids in their comprehension of actual expenses and utilization in a real-world setting.
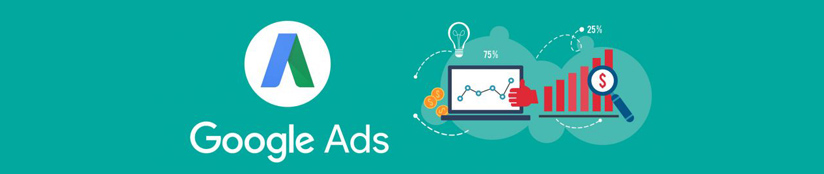
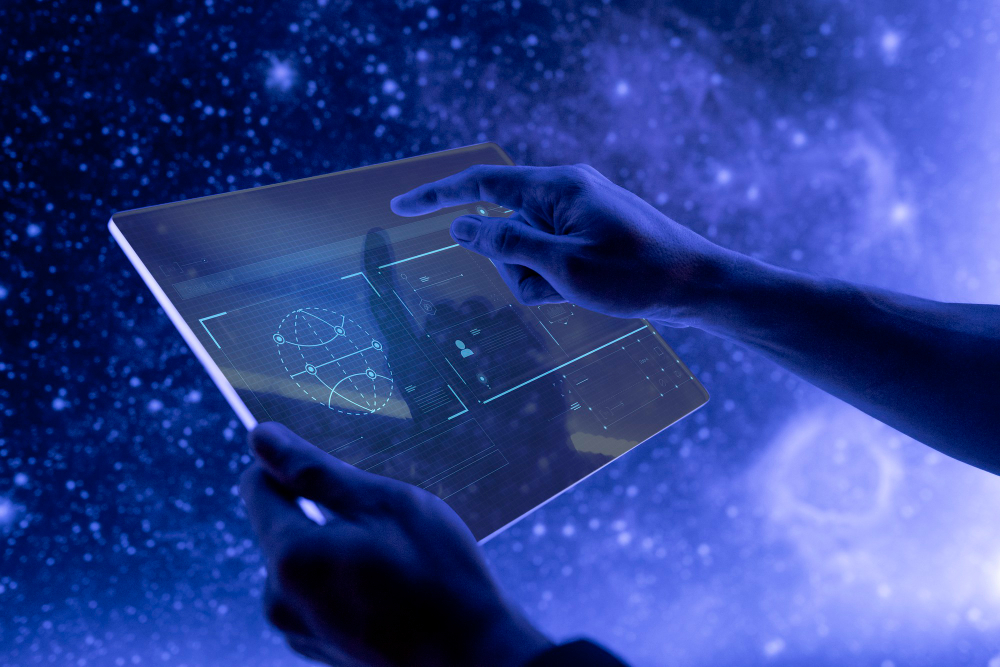
The paradox of productivity
Although AI has the potential to increase productivity, it can be difficult to convert these gains into quantifiable financial gains. As Microsoft Copilot and other such solutions show, saving time alone does not automatically translate into increased revenue or decreased expenses, according to Suda.
“It’s imperative to have a clear understanding of productivity and how to convert its benefits into value, be it increased revenue or decreased expenses,” Suda stated.
He also emphasized the significance of differentiating between value and advantages. Although benefits like faster processing times, better customer experiences, and higher productivity are important, they only become valuable when they promote profitability.
Generative AI, for example, may expedite the time needed for a series of professional services, but this efficiency is not indicative of AI delivering on its promised value until it is translated into increased revenue or decreased costs.
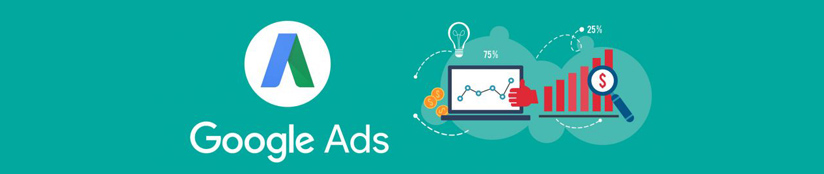
The risk of cost overruns
Suda also brought up the important aspect of the possibility of cost overruns brought on by unforeseen user behavior. The expenses that emerge from an AI system that becomes extremely popular and is used more than anticipated can be enormous. This hypothetical situation emphasizes how crucial it is to carefully plan ahead and monitor AI deployments in real time in order to efficiently control and forecast costs.
“Your costs can go through the roof if users love the AI and use it extensively,” Suda stated. “That’s why it’s so important to comprehend and model user behavior.”
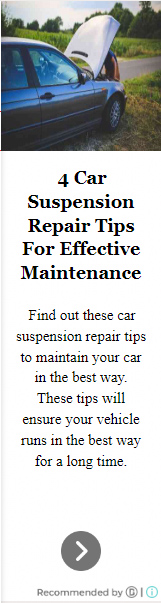